Building machines that work for everyone – how diversity of test subjects is a technology blind spot, and what to do about it
- Written by Tahira Reid, Associate Professor of Mechanical Engineering, Purdue University
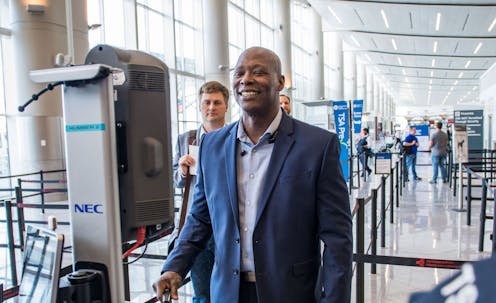
People interact with machines in countless ways every day. In some cases, they actively control a device, like driving a car or using an app on a smartphone. Sometimes people passively interact with a device, like being imaged by an MRI machine. And sometimes they interact with machines without consent or even knowing about the interaction, like being scanned by a law enforcement facial recognition system.
Human-Machine Interaction (HMI) is an umbrella term that describes the ways people interact with machines. HMI is a key aspect of researching, designing and building new technologies, and also studying how people use and are affected by technologies.
Researchers, especially those traditionally trained in engineering, are increasingly taking a human-centered approach when developing systems and devices. This means striving to make technology that works as expected for the people who will use it by taking into account what’s known about the people and by testing the technology with them. But even as engineering researchers increasingly prioritize these considerations, some in the field have a blind spot: diversity.
As an interdisciplinary researcher[1] who thinks holistically about engineering and design and an expert in dynamics and smart materials[2] with interests in policy, we have examined the lack of inclusion[3] in technology design, the negative consequences and possible solutions.
People at hand
Researchers and developers typically follow a design process that involves testing key functions and features before releasing products to the public. Done properly, these tests can be a key component of compassionate design[4]. The tests can include interviews and experiments with groups of people who stand in for the public.
Tahira Reid discusses compassionate design in engineering.In academic settings, for example, the majority of study participants are students. Some researchers attempt to recruit off-campus participants, but these communities are often similar to the university population. Coffee shops and other locally owned businesses, for example, may allow flyers to be posted in their establishments. However, the clientele of these establishments is often students, faculty and academic staff.
In many industries, co-workers serve as test participants for early-stage work because it is convenient to recruit from within a company. It takes effort to bring in outside participants, and when they are used, they often reflect the majority population. Therefore, many of the people who participate in these studies have similar demographic characteristics.
Real-world harm
It is possible to use a homogenous sample of people in publishing a research paper that adds to a field’s body of knowledge. And some researchers who conduct studies this way acknowledge the limitations of homogenous study populations. However, when it comes to developing systems that rely on algorithms, such oversights can cause real-world problems[5]. Algorithms are as only as good as the data that is used to build them.
Algorithms are often based on mathematical models that capture patterns and then inform a computer about those patterns to perform a given task. Imagine an algorithm designed to detect when colors appear on a clear surface. If the set of images used to train that algorithm consists of mostly shades of red, the algorithm might not detect when a shade of blue or yellow is present.
In practice, algorithms have failed to detect darker skin tones for Google’s skincare program[6] and in automatic soap dispensers[7]; accurately identify a suspect, which led to the wrongful arrest of an innocent man in Detroit[8]; and reliably identify women of color[9]. MIT artificial intelligence researcher Joy Buolamwini describes this as algorithmic bias and has extensively discussed and published work on these issues[10].
Many problems, like this automated soap dispenser working for a white hand but not a Black hand, could be avoided with more inclusive testing.Even as the U.S. fights COVID-19, the lack of diverse training data has become evident in medical devices. Pulse oximeters, which are essential for keeping track of your health at home and to indicate when you might need hospitalization, may be less accurate for people with melanated skin[11]. These design flaws, like those in algorithms, are not inherent to the device[12] but can be traced back to the technology being designed and tested using populations that were not diverse enough to represent all potential users.
Being inclusive
Researchers in academia are often under pressure to publish research findings as quickly as possible. Therefore, reliance on convenience samples[13] – that is, people who are easy to reach and get data from – is very common.
Though institutional review boards[14] exist to ensure that study participants’ rights are protected and that researchers follow proper ethics in their work, they don’t have the responsibility to dictate to researchers who they should recruit. When researchers are pressed for time, considering different populations for study subjects can mean additional delay. Finally, some researchers may simply be unaware of how to adequately diversify their study’s subjects.
There are several ways researchers in academia and industry can increase the diversity of their study participant pools.
One is to make time to do the inconvenient and sometimes hard work of developing inclusive recruitment strategies. This can require creative thinking. One such method is to recruit diverse students who can serve as ambassadors[15] to diverse communities. The students can gain research experience while also serving as a bridge between their communities and researchers.
Another is to allow members of the community to participate in the research and provide consent for new and unfamiliar technologies whenever possible. For example, research teams can form an advisory board composed of members from various communities. Some fields frequently include an advisory board as part of their government-funded research plans.
Another approach is to include people who know how to think through cultural implications of technologies as members of the research team. For instance, the New York City Police Department’s use of a robotic dog[16] in Brooklyn, Queens and the Bronx sparked outrage among residents. This might have been avoided if they had engaged with experts in the social sciences or science and technology studies, or simply consulted with community leaders.
[Over 140,000 readers rely on The Conversation’s newsletters to understand the world. Sign up today[17].]
Lastly, diversity is not just about race but also age, gender identity, cultural backgrounds, educational levels, disability, English proficiency and even socioeconomic levels. Lyft is on a mission to deploy robotaxis next year[18], and experts are excited about the prospects of using robotaxis to transport the elderly and disabled[19]. It is not clear whether these aspirations include those who live in less-affluent or low-income communities, or lack the family support that could help prepare people to use the service. Before dispatching a robotaxi to transport grandmothers, it’s important to take into account how a diverse range of people will experience the technology.
References
- ^ interdisciplinary researcher (scholar.google.com)
- ^ expert in dynamics and smart materials (scholar.google.com)
- ^ examined the lack of inclusion (doi.org)
- ^ compassionate design (doi.org)
- ^ can cause real-world problems (theconversation.com)
- ^ Google’s skincare program (www.euronews.com)
- ^ automatic soap dispensers (reporter.rit.edu)
- ^ wrongful arrest of an innocent man in Detroit (www.nytimes.com)
- ^ reliably identify women of color (www.nist.gov)
- ^ discussed and published work on these issues (www.ajl.org)
- ^ melanated skin (dx.doi.org)
- ^ inherent to the device (dx.doi.org)
- ^ convenience samples (doi.org)
- ^ institutional review boards (dx.doi.org)
- ^ recruit diverse students who can serve as ambassadors (dx.doi.org)
- ^ use of a robotic dog (www.nytimes.com)
- ^ Sign up today (memberservices.theconversation.com)
- ^ Lyft is on a mission to deploy robotaxis next year (www.lyft.com)
- ^ transport the elderly and disabled (www.techrepublic.com)
Authors: Tahira Reid, Associate Professor of Mechanical Engineering, Purdue University