Does your AI discriminate?
- Written by Julie Manning Magid, Professor of Business Law, IUPUI
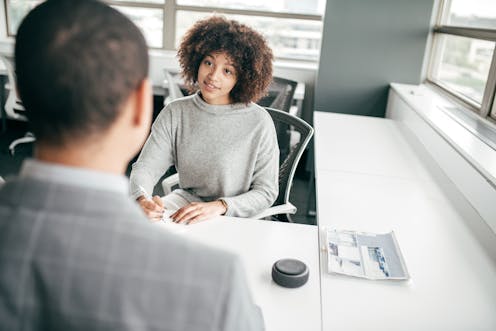
Women leaders like New Zealand Prime Minister Jacinda Ardern[1] and San Francisco Mayor London Breed[2] are receiving recognition for their quick action[3] in the face of the COVID-19 pandemic.
But men are chosen as leaders of government around the world in vastly greater numbers[4].
This disparity is not confined to political leadership. In 2019, Forbes choose 100 of America’s “Most Influential Leaders,” and 99 of them were men[5].
The lack of diversity is not limited to gender. A survey of nonprofit sector[6] chief executives found that 87% of survey respondents self-identified as white.
As the executive and academic director of a leadership center[7], I study employment discrimination and inclusion[8]. I’ve seen that many organizations want a process where bias could be removed[9] from identifying leaders. Investors want to invest in businesses[10] with diverse workforces, and employees want to work in diverse organizations[11].
My research[12] indicates that relying on data analytics to eliminate human bias in choosing leaders won’t help.
AI isn’t foolproof
Employers increasingly rely[13] on algorithms to determine who advances through application portals to an interview.
As labor rights scholar Ifeoma Ajunwa[14] writes, “Algorithmic decision-making is the civil rights issue of the 21st century.” In February 2020, the U.S. House of Representatives’ Committee on Education and Labor convened a hearing called “The Future of Work: Protecting Workers’ Civil Rights in the Digital Age[15].”
Hiring algorithms create a selection process that offers no transparency and is not monitored. Applicants struck from an application process – or as Ajunwa refers to it, “algorithmically blackballed[16]” – have few legal protections.
For instance, in 2014, Amazon reportedly[17] began developing a computer-based program to identify the best resumes submitted for jobs. The idea was to automate a process and gain efficiency, much as it has done with other aspects of its business.
However, by using computer models to observe patterns in the previous 10 years of submitted resumes to choose the best, the computer taught itself that resumes from men were preferred to a resume that included the word “women’s,” as in a women’s club or organization. Amazon subsequently abandoned the project, according to reports[18].
Although often historic biases[19] are inadvertently built into algorithms and reflect human prejudices, recent scholarship[20] by Philip M. Nichols has identified an additional threat of potential intentional manipulation of underlying algorithms to benefit third parties.
Inadvertent or intentional, the ability to detect bias of an algorithm is extremely difficult because it can occur at any stage[21] of the development of AI, from data collection to modeling.
Therefore, although organizations have access to leadership analytical tools[22] based on research and analysis of leadership traits[23], the white male leader stereotype is deeply ingrained and even sometimes perpetuated[24] by those who themselves are diverse. This cannot be eliminated simply by developing an algorithm that selects leaders.
After the interviews
The data to build these algorithms increase exponentially.
One video interview service[25], HireVue, boasts of its ability to detect thousands of data points in a single 30-minute interview, from sentence structure to facial movements, to determine employability against other applicants.
Imagine the opportunity, then, for a current employer to collect data continuously to determine leadership potential and promotions of its workforce. For instance, cameras in the workplace[26] can collect facial expressions all day at work, particularly when entering and exiting the workplace.
Increasingly, the data are not just collected during the work day or while at work, but during off-duty conduct as well. In a recent article[27], Professor Leora Eisenstaedt identified workplace programs that gathered massive amounts of data of off-duty conduct of employees from Facebook posts and Fitbit usage, for example, without transparency about future use of the data. Employers then used those bits of data to draw correlations to predict workplace success.
As Eisenstaedt notes, most workers “will likely chafe at the notion that their taste in beer, love of indie rock and preference for the Washington Post, along with thousands of other variables, can be used to determine professional development opportunities, leadership potential and future career success.”
Nonetheless, that potential exists today in workplaces, and the law simply has not caught up to the vast amount of data collected and utilized by employers wanting to know the promotion and leadership investment in its employees is supported by the data.
In many cases, employees agree to collection of meta-data without a thorough understanding of what that data can reveal and how it can be used to help or hamper a career.
[You’re smart and curious about the world. So are The Conversation’s authors and editors. You can read us daily by subscribing to our newsletter[28].]
References
- ^ Jacinda Ardern (www.theatlantic.com)
- ^ London Breed (www.theatlantic.com)
- ^ quick action (www.forbes.com)
- ^ as leaders of government around the world in vastly greater numbers (www.unwomen.org)
- ^ 99 of them were men (www.businessinsider.com)
- ^ survey of nonprofit sector (www.battaliawinston.com)
- ^ leadership center (tobiascenter.iu.edu)
- ^ employment discrimination and inclusion (kelley.iu.edu)
- ^ bias could be removed (www.blackrock.com)
- ^ Investors want to invest in businesses (www.gsb.stanford.edu)
- ^ employees want to work in diverse organizations (hrexecutive.com)
- ^ research (home.kelley.iupui.edu)
- ^ increasingly rely (www.nytimes.com)
- ^ Ifeoma Ajunwa (news.cornell.edu)
- ^ The Future of Work: Protecting Workers’ Civil Rights in the Digital Age (docs.house.gov)
- ^ algorithmically blackballed (www.shrm.org)
- ^ Amazon reportedly (www.reuters.com)
- ^ reports (www.reuters.com)
- ^ historic biases (www.princeton.edu)
- ^ recent scholarship (doi.org)
- ^ stage (towardsdatascience.com)
- ^ analytical tools (www.hrtechnologist.com)
- ^ leadership traits (www.inc.com)
- ^ perpetuated (www.scientificamerican.com)
- ^ video interview service (www.washingtonpost.com)
- ^ cameras in the workplace (employment.findlaw.com)
- ^ recent article (doi.org)
- ^ You can read us daily by subscribing to our newsletter (theconversation.com)
Authors: Julie Manning Magid, Professor of Business Law, IUPUI
Read more https://theconversation.com/does-your-ai-discriminate-132847