Examining a video's changes over time helps flag deepfakes
- Written by Wael Abd-Almageed, Research Team Lead and Senior Scientist, Information Sciences Institute, University of Southern California
It used to be that only Hollywood production companies with deep pockets and teams of skilled artists and technicians could make deepfake videos, realistic fabrications appearing to show people doing and saying things they never actually did or said. Not anymore – software freely available online lets anyone with a computer and some time on their hands create convincing fake videos.
Whether used for personal revenge, to harass celebrities or to influence public opinion, deepfakes render untrue the age-old axiom that “seeing is believing.”
My research team[1] and I at the University of Southern California Information Sciences Institute are developing ways to tell the difference between realistic-looking fakes and genuine videos that show actual events as they happened. Our recent research has found a new and apparently more accurate way[2] to detect deepfake videos.
Barack Obama would never say these things – and never did.Finding the flaws
Generally speaking, various deepfake algorithms work by using machine learning techniques to analyze images of the target, identifying key elements of facial expressions like the nose, corners of the mouth, locations of the eyes and so on. They use that information to synthesize new images of that person’s face and put them together to create a video of the target that looks realistic but is fake.
Most current methods of detecting deepfakes involve looking at each frame of a video separately, either manually or using a detection algorithm[3], to spot tiny flaws left by the image-fabrication process[4]. If there is enough evidence of tampering in enough frames, the video is considered fake.
However, deepfake creators have begun to use large amounts of image and video compression[5] to blur their results, hiding any artifacts that might reveal their falsehood.
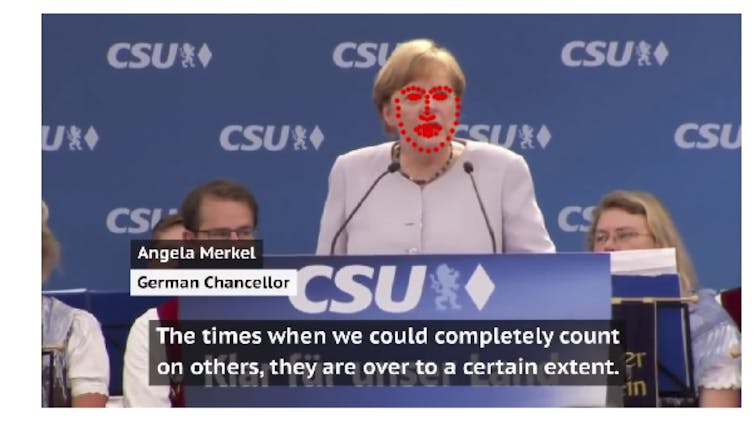
Looking at sequences, not single frames
Our method seeks to get around that deception by taking a different approach. We extract all the frames from a video and identify the areas that show the target’s face. Then we, in effect, stack all those face images on top of each other, making sure the nose, eyes and mouth are all aligned between every frame. This eliminates the effects of head movements or camera-angle shifts in the video.
Then, rather than looking at each face image individually, we look for inconsistencies in how different parts of the face move from frame to frame over time. It’s sort of like setting up a kids’ flip-book and then watching for weird jumps in the sequence. We have found that this method is more accurate[7], in part because we can identify more evidence of falsehood than when looking at each frame alone.
The red areas are where the algorithm detects inconsistencies, suggesting the video is fake.Specifically, we detected deepfakes 96% of the time[8], even when the images and videos are significantly compressed. So far we have found that level of accuracy only on the only large-scale database available to academic researchers for evaluating their deepfake detection techniques, which is called FaceForensics++[9]. That data set contains videos from three of the most prominent deepfake-generation algorithms, Face2Face, FaceSwap and DeepFake, though fakers are always improving their methods.
Deepfake detection is an arms race, in which fakers and truth-seekers will keep advancing their respective technologies. Therefore, the job of limiting their effects on society as a whole can’t fall only to researchers. Scholars and experimenters must keep working, of course, but that’s not all. I believe social networking platforms should also work to develop software and policies that slow the spread of misinformation of all types – whether manipulating a person’s face or showing their whole body[10] moving in ways they never could.
References
- ^ My research team (www.isi.edu)
- ^ new and apparently more accurate way (openaccess.thecvf.com)
- ^ using a detection algorithm (theconversation.com)
- ^ tiny flaws left by the image-fabrication process (theconversation.com)
- ^ use large amounts of image and video compression (ieeexplore.ieee.org)
- ^ CC BY-ND (creativecommons.org)
- ^ this method is more accurate (openaccess.thecvf.com)
- ^ detected deepfakes 96% of the time (openaccess.thecvf.com)
- ^ FaceForensics++ (arxiv.org)
- ^ showing their whole body (arxiv.org)
Authors: Wael Abd-Almageed, Research Team Lead and Senior Scientist, Information Sciences Institute, University of Southern California
Read more http://theconversation.com/examining-a-videos-changes-over-time-helps-flag-deepfakes-120263