Is it the end of 'statistical significance'? The battle to make science more uncertain
- Written by Valen E. Johnson, University Distinguished Professor and Department Head of Statistics, Texas A&M University
The scientific world is abuzz following recommendations by two of the most prestigious scholarly journals – The American Statistician[1] and Nature[2] – that the term “statistical significance[3]” be retired.
In their introduction to the special issue of The American Statistician on the topic, the journal’s editors urge “moving to a world beyond ‘p<0.05,’” the famous 5 percent threshold[4] for determining whether a study’s result is statistically significant. If a study passes this test, it means that the probability of a result being due to chance alone is less than 5 percent. This has often been understood to mean that the study is worth paying attention to.
The journal’s basic message – but not necessarily the consensus of the 43 articles in this issue, one of which I contributed[5] – was that scientists first and foremost should “embrace uncertainty” and “be thoughtful, open and modest.”
While these are fine qualities, I believe that scientists must not let them obscure the precision and rigor that science demands. Uncertainty is inherent in data. If scientists further weaken the already very weak threshold of 0.05, then that would inevitably make scientific findings more difficult to interpret and less likely to be trusted.
Piling difficulty on top of difficulty
In the traditional practice of science, a scientist generates a hypothesis and designs experiments to collect data in support of hypotheses. He or she then collects data and performs statistical analyses to determine if the data did in fact support the hypothesis.
One standard statistical analysis is the p-value[6]. This generates a number between 0 and 1 that indicates strong, marginal or weak support of a hypothesis.
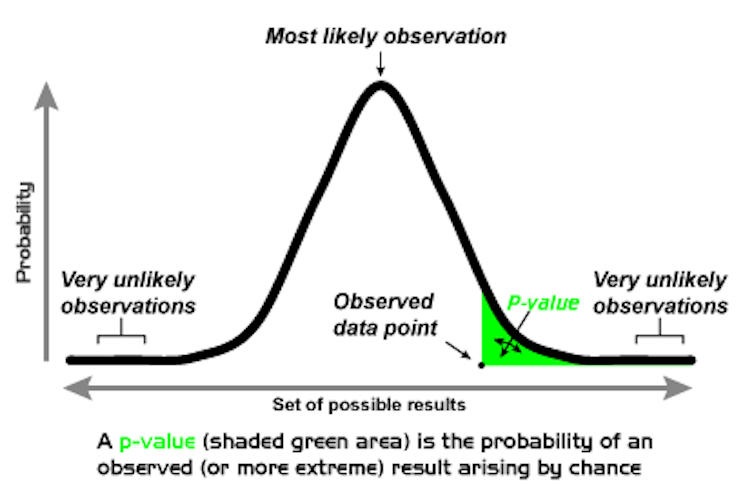
But I worry that abandoning evidence-driven standards for these judgments will make it even more difficult to design experiments, much less assess their outcomes. For instance, how could one even determine an appropriate sample size without a targeted level of precision? And how are research results to be interpreted?
These are important questions, not just for researchers at funding or regulatory agencies, but for anyone whose daily life is influenced by statistical judgments. That includes anyone who takes medicine or undergoes surgery, drives or rides in vehicles, is invested in the stock market, has life insurance or depends on accurate weather forecasts… and the list goes on. Similarly, many regulatory agencies rely on statistics to make decisions every day.
Scientists must have the language to indicate that a study, or group of studies, provided significant evidence in favor of a relationship or an effect. Statistical significance is the term that serves this purpose.
The groups behind this movement
Hostility to the term “statistical significance” arises from two groups.
The first is largely made up of scientists disappointed when their studies produce p=0.06. In other words, those whose studies just don’t make the cut. These are largely scientists who find the 0.05 standard too high[8] a hurdle for getting published in the scholarly journals that are a major source of academic knowledge – as well as tenure and promotion.
The second group is concerned over the failure to replicate scientific studies[9], and they blame significance testing in part for this failure.
For example, a group of scientists[10] recently repeated 100 published psychology experiments. Ninety-seven of the 100 original studies reported a statistically significant finding (p<0.05), but only 36 of the repeated experiments were able to also achieving a significant result.
The failure of so many studies to replicate can be partially blamed on publication bias, which results when only significant findings are published. Publication bias causes scientists to overestimate the magnitude of an effect[11], such as the relationship between two variables, making replication less likely.
Complicating the situation even further is the fact that recent research[12] shows that the p-value cutoff doesn’t provide much evidence that a real relationship has been found. In fact, in replication studies in social sciences, it now appears that p-values close to the standard threshold of 0.05 probably mean that a scientific claim is wrong. It’s only when the p-value is much smaller, maybe less than 0.005, that scientific claims are likely to show a real relationship[13].
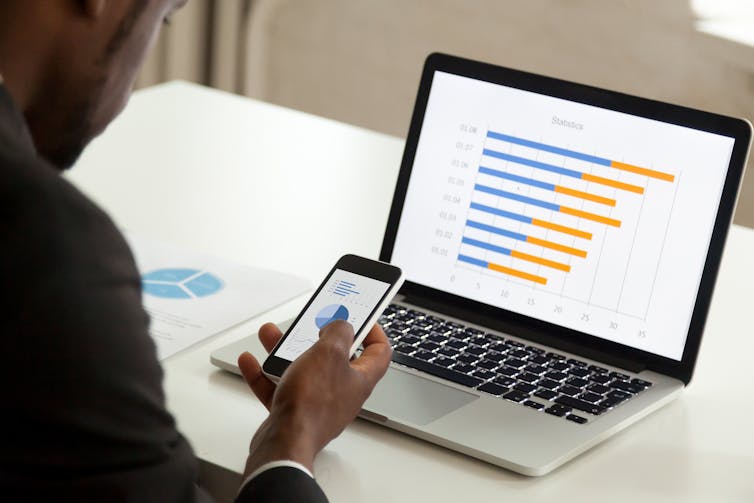
The confusion leading to this movement
Many nonstatisticians confuse p-value with the probability[15] that no discovery was made.
Let’s look at an example from the Nature article. Two studies[16] examined the increased risk of disease after taking a drug. Both studies estimated that patients had a 20 percent higher risk of getting the disease if they take the drug than if they didn’t. In other words, both studies estimated the relative risk[17] to be 1.20.
However, the relative risk estimated from one study was more precise than the other, because its estimate was based on outcomes from many more patients. Thus, the estimate from one study was statistically significant, and the estimate from the other was not.
The authors cite this inconsistency – that one study obtained a significant result and the other didn’t – as evidence that statistical significance leads to misinterpretation of scientific results.
However, I feel that a reasonable summary is simply that one study collected statistically significant evidence and one did not, but the estimates from both studies suggested that relative risk was near 1.2.
Where to go from here
I agree with the Nature article and The American Statistician editorial that data collected from all well-designed scientific studies should be made publicly available, with comprehensive summaries of statistical analyses. Along with each study’s p-values, it is important to publish estimates of effect sizes and confidence intervals for these estimates, as well as complete descriptions of all data analyses and data processing.
On the other hand, only studies that provide strong evidence in favor of important associations or new effects should be published in premier journals. For these journals, standards of evidence should be increased by requiring smaller p-values for the initial report of relationships and new discoveries. In other words, make scientists publish results that they’re even more certain about.
The bottom line is that dismantling accepted standards of statistical evidence will decrease the uncertainty that scientists have in publishing their own research. But it will also increase the public’s uncertainty in accepting the findings that they do publish – and that can be problematic.
References
- ^ The American Statistician (www.tandfonline.com)
- ^ Nature (www.nature.com)
- ^ statistical significance (en.wikipedia.org)
- ^ the famous 5 percent threshold (www.haghish.com)
- ^ I contributed (doi.org)
- ^ p-value (en.wikipedia.org)
- ^ CC BY-SA (creativecommons.org)
- ^ scientists who find the 0.05 standard too high (www.researchgate.net)
- ^ failure to replicate scientific studies (theconversation.com)
- ^ a group of scientists (doi.org)
- ^ magnitude of an effect (doi.org)
- ^ recent research (doi.org)
- ^ show a real relationship (www.nature.com)
- ^ fizkes/shutterstock.com (www.shutterstock.com)
- ^ probability (en.wikipedia.org)
- ^ Two studies (doi.org)
- ^ relative risk (en.wikipedia.org)
Authors: Valen E. Johnson, University Distinguished Professor and Department Head of Statistics, Texas A&M University