Machine learning cracked the protein-folding problem and won the 2024 Nobel Prize in chemistry
- Written by Marc Zimmer, Professor of Chemistry, Connecticut College
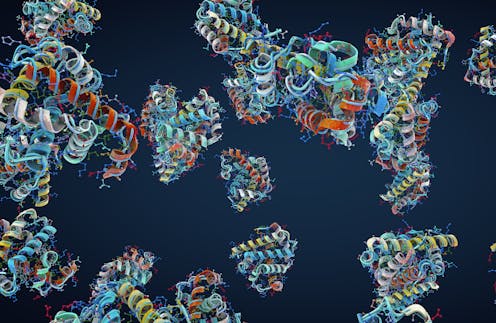
The 2024 Nobel Prize in chemistry[1] recognized Demis Hassabis[2], John Jumper[3] and David Baker[4] for using machine learning to tackle one of biology’s biggest challenges: predicting the 3D shape of proteins[5] and designing them from scratch.
This year’s award stood out because it honored research that originated at a tech company: DeepMind, an AI research startup that was acquired by Google in 2014[6]. Most previous chemistry Nobel Prizes have gone to researchers in academia. Many laureates went on to form startup companies to further expand and commercialize their groundbreaking work – for instance, CRISPR gene-editing technology[7] and quantum dots[8] – but the research, from start to end, wasn’t done in the commercial sphere.
Although the Nobel Prizes in physics and chemistry are awarded separately, there is a fascinating connection between the winning research in those fields in 2024. The physics award went to two computer scientists[9] who laid the foundations for machine learning[10], while the chemistry laureates were rewarded for their use of machine learning to tackle one of biology’s biggest mysteries: how proteins fold.
The 2024 Nobel Prizes underscore both the importance of this kind of artificial intelligence and how science today often crosses traditional boundaries, blending different fields to achieve groundbreaking results.
The challenge of protein folding
Proteins are the molecular machines of life. They make up a significant portion of our bodies, including muscles, enzymes, hormones, blood, hair and cartilage.
Understanding proteins’ structures is essential because their shapes determine their functions. Back in 1972, Christian Anfinsen won the Nobel Prize[12] in chemistry for showing that the sequence of a protein’s amino acid building blocks dictates the protein’s shape[13], which, in turn, influences its function. If a protein folds incorrectly, it may not work properly and could lead to diseases such as Alzheimer’s[14], cystic fibrosis[15] or diabetes[16].
A protein’s overall shape depends on the tiny interactions, the attractions and repulsions, between all the atoms in the amino acids its made of. Some want to be together, some don’t. The protein twists and folds itself into a final shape based on many thousands of these chemical interactions.
For decades, one of biology’s greatest challenges was predicting a protein’s shape based solely on its amino acid sequence. Although researchers can now predict the shape, we still don’t understand how the proteins maneuver into their specific shapes and minimize the repulsions of all the interatomic interactions in a few microseconds.
To understand how proteins work and to prevent misfolding, scientists needed a way to predict the way proteins fold, but solving this puzzle was no easy task.
In 2003, University of Washington biochemist David Baker[17] wrote Rosetta[18], a computer program for designing proteins. With it he showed it was possible to reverse the protein-folding problem by designing a protein shape and then predicting[19] the amino acid sequence needed to create it.
It was a phenomenal jump forward, but the shape chosen for the calculation was simple, and the calculations were complex. A major paradigm shift was required to routinely design novel proteins with desired structures.
A new era of machine learning
Machine learning is a type of AI where computers learn to solve problems by analyzing vast amounts of data. It’s been used in various fields, from game-playing[20] and speech recognition[21] to autonomous vehicles[22] and scientific research[23]. The idea behind machine learning is to use hidden patterns in data to answer complex questions.
This approach made a huge leap in 2010 when Demis Hassabis co-founded DeepMind[24], a company aiming to combine neuroscience with AI to solve real-world problems.
Hassabis, a chess prodigy at age 4, quickly made headlines with AlphaZero[25], an AI that taught itself to play chess at a superhuman level. In 2017, AlphaZero thoroughly beat the world’s top computer chess program, Stockfish-8. The AI’s ability to learn from its own gameplay, rather than relying on preprogrammed strategies, marked a turning point in the AI world.
Soon after, DeepMind applied similar techniques to Go, an ancient board game known for its immense complexity. In 2016, its AI program AlphaGo[26] defeated one of the world’s top players, Lee Sedol, in a widely watched match that stunned millions[27].
In 2016, Hassabis shifted DeepMind’s focus to a new challenge: the protein-folding problem. Under the leadership of John Jumper[29], a chemist with a background in protein science, the AlphaFold project began. The team used a large database of experimentally determined protein structures to train the AI, which allowed it to learn the principles of protein folding. The result was AlphaFold2[30], an AI that could predict the 3D structure of proteins from their amino acid sequences with remarkable accuracy.
This was a significant scientific breakthrough. AlphaFold has since predicted the structures of over 200 million proteins – essentially all the proteins that scientists have sequenced to date. This massive database of protein structures[31] is now freely available, accelerating research in biology, medicine and drug development.
Designer proteins to fight disease
Understanding how proteins fold and function is crucial for designing new drugs. Enzymes[32], a type of protein, act as catalysts in biochemical reactions and can speed up or regulate these processes. To treat diseases such as cancer or diabetes, researchers often target specific enzymes involved in disease pathways. By predicting the shape of a protein, scientists can figure out where small molecules – potential drug candidates – might bind to it, which is the first step in designing new medicines[33].
In 2024, DeepMind launched AlphaFold3[34], an upgraded version of the AlphaFold program that not only predicts protein shapes but also identifies potential binding sites for small molecules. This advance makes it easier for researchers to design drugs that precisely target the right proteins.
Google bought Deepmind[35] for reportedly around half a billion dollars in 2014[36]. Google DeepMind has now started a new venture, Isomorphic Labs[37], to collaborate with pharmaceutical companies on real-world drug development using these AlphaFold3 predictions.
For his part, David Baker has continued to make significant contributions to protein science. His team at the University of Washington developed an AI-based method called “family-wide hallucination[38],” which they used to design entirely new proteins from scratch. Hallucinations are new patterns – in this case, proteins – that are plausible, meaning they are a good fit with patterns in the AI’s training data. These new proteins included a light-emitting enzyme, demonstrating that machine learning can help create novel synthetic proteins. These AI tools offer new ways to design functional enzymes and other proteins that never could have evolved naturally.
AI will enable research’s next chapter
The Nobel-worthy achievements of Hassabis, Jumper and Baker show that machine learning isn’t just a tool for computer scientists – it’s now an essential part of the future of biology and medicine.
By tackling one of the toughest problems in biology, the winners of the 2024 prize have opened up new possibilities in drug discovery, personalized medicine and even our understanding of the chemistry of life itself.
References
- ^ 2024 Nobel Prize in chemistry (www.nobelprize.org)
- ^ Demis Hassabis (www.nobelprize.org)
- ^ John Jumper (scholar.google.com)
- ^ David Baker (scholar.google.com)
- ^ 3D shape of proteins (www.nobelprize.org)
- ^ Google in 2014 (www.theguardian.com)
- ^ CRISPR gene-editing technology (www.invent.org)
- ^ quantum dots (lumicell.com)
- ^ went to two computer scientists (www.nobelprize.org)
- ^ laid the foundations for machine learning (theconversation.com)
- ^ ©Johan Jarnestad/The Royal Swedish Academy of Sciences (www.nobelprize.org)
- ^ Christian Anfinsen won the Nobel Prize (www.nobelprize.org)
- ^ dictates the protein’s shape (www.nature.com)
- ^ Alzheimer’s (www.bmglabtech.com)
- ^ cystic fibrosis (doi.org)
- ^ diabetes (elifesciences.org)
- ^ David Baker (www.bakerlab.org)
- ^ Rosetta (docs.rosettacommons.org)
- ^ designing a protein shape and then predicting (doi.org)
- ^ game-playing (www.nytimes.com)
- ^ speech recognition (aiola.com)
- ^ autonomous vehicles (www.axios.com)
- ^ scientific research (doi.org)
- ^ DeepMind (deepmind.google)
- ^ AlphaZero (deepmind.google)
- ^ AlphaGo (doi.org)
- ^ widely watched match that stunned millions (www.theatlantic.com)
- ^ AP Photo/Alastair Grant (newsroom.ap.org)
- ^ John Jumper (www.nobelprize.org)
- ^ AlphaFold2 (theconversation.com)
- ^ massive database of protein structures (alphafold.ebi.ac.uk)
- ^ Enzymes (www.britannica.com)
- ^ designing new medicines (theconversation.com)
- ^ AlphaFold3 (blog.google)
- ^ Google bought Deepmind (archive.nytimes.com)
- ^ half a billion dollars in 2014 (www.theguardian.com)
- ^ Isomorphic Labs (www.isomorphiclabs.com)
- ^ family-wide hallucination (doi.org)
Authors: Marc Zimmer, Professor of Chemistry, Connecticut College