How foreign operations are manipulating social media to influence your views
- Written by Filippo Menczer, Professor of Informatics and Computer Science, Indiana University
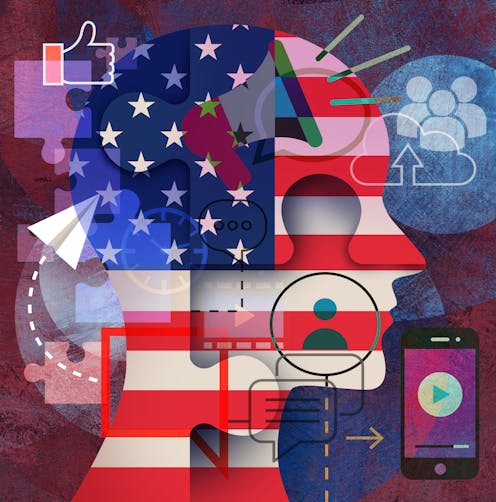
Foreign influence campaigns, or information operations, have been widespread[1] in the run-up to the 2024 U.S. presidential election. Influence campaigns are large-scale efforts to shift public opinion, push false narratives or change behaviors among a target population. Russia[2], China[3], Iran[4], Israel[5] and other nations have run these campaigns by exploiting social bots[6], influencers[7], media companies[8] and generative AI[9].
At the Indiana University Observatory on Social Media[10], my colleagues and I[11] study influence campaigns and design technical solutions – algorithms – to detect[12] and counter them. State-of-the-art methods[13] developed in our center use several indicators of this type of online activity, which researchers call inauthentic coordinated behavior. We identify clusters of social media accounts that post in a synchronized fashion, amplify the same groups of users, share identical sets of links, images or hashtags, or perform suspiciously similar sequences of actions[14].
We have uncovered many examples of coordinated inauthentic behavior. For example, we found accounts that flood the network with tens or hundreds of thousands of posts in a single day. The same campaign can post a message with one account and then have other accounts that its organizers also control “like” and “unlike” it hundreds of times in a short time span. Once the campaign achieves its objective, all these messages can be deleted to evade detection[15]. Using these tricks, foreign governments and their agents can manipulate social media algorithms that determine what is trending and what is engaging to decide what users see in their feeds.
Adversaries such as Russia, China and Iran aren’t the only foreign governments manipulating social media to influence U.S. politics.Generative AI
One technique increasingly being used is creating and managing armies of fake accounts with generative artificial intelligence. We analyzed 1,420 fake Twitter – now X – accounts that used AI-generated faces[16] for their profile pictures. These accounts were used to spread scams, disseminate spam and amplify coordinated messages, among other activities.
We estimate that at least 10,000 accounts like these were active daily on the platform, and that was before X CEO Elon Musk dramatically cut the platform’s trust and safety teams[17]. We also identified a network of 1,140 bots that used ChatGPT[18] to generate humanlike content to promote fake news websites and cryptocurrency scams.
In addition to posting machine-generated content, harmful comments and stolen images, these bots engaged with each other and with humans through replies and retweets. Current state-of-the-art large language model content detectors are unable to distinguish[19] between AI-enabled social bots and human accounts in the wild.
Model misbehavior
The consequences of such operations are difficult to evaluate due to the challenges posed by collecting[20] data[21] and carrying out ethical experiments[22] that would influence online communities. Therefore it is unclear, for example, whether online influence campaigns can sway election outcomes[23]. Yet, it is vital to understand society’s vulnerability to different manipulation tactics.
In a recent paper, we introduced a social media model called SimSoM that simulates how information spreads through the social network[24]. The model has the key ingredients of platforms such as Instagram, X, Threads, Bluesky and Mastodon: an empirical follower network, a feed algorithm, sharing and resharing mechanisms, and metrics for content quality, appeal and engagement.
SimSoM allows researchers to explore scenarios in which the network is manipulated by malicious agents who control inauthentic accounts. These bad actors aim to spread low-quality information, such as disinformation, conspiracy theories, malware or other harmful messages. We can estimate the effects of adversarial manipulation tactics by measuring the quality of information that targeted users are exposed to in the network.
We simulated scenarios to evaluate the effect of three manipulation tactics. First, infiltration: having fake accounts create believable interactions with human users in a target community, getting those users to follow them. Second, deception: having the fake accounts post engaging content, likely to be reshared by the target users. Bots can do this by, for example, leveraging emotional responses and political alignment. Third, flooding: posting high volumes of content.
Our model shows that infiltration is the most effective tactic, reducing the average quality of content in the system by more than 50%. Such harm can be further compounded by flooding the network with low-quality yet appealing content, thus reducing quality by 70%.
Curbing coordinated manipulation
We have observed all these tactics in the wild. Of particular concern is that generative AI models can make it much easier and cheaper for malicious agents to create and manage believable accounts. Further, they can use generative AI to interact nonstop with humans and create and post harmful but engaging content on a wide scale. All these capabilities are being used to infiltrate social media users’ networks and flood their feeds with deceptive posts.
These insights suggest that social media platforms should engage in more – not less – content moderation[25] to identify and hinder manipulation campaigns and thereby increase their users’ resilience to the campaigns.
The platforms can do this by making it more difficult for malicious agents to create fake accounts and to post automatically. They can also challenge accounts that post at very high rates to prove that they are human. They can add friction[26] in combination with educational efforts, such as nudging users to reshare accurate information[27]. And they can educate users about their vulnerability to deceptive AI-generated content.
Open-source AI models and data make it possible for malicious agents to build their own generative AI tools. Regulation should therefore target AI content dissemination[28] via social media platforms rather then AI content generation. For instance, before a large number of people can be exposed to some content, a platform could require its creator to prove its accuracy or provenance.
These types of content moderation would protect, rather than censor, free speech[29] in the modern public squares. The right of free speech is not a right of exposure, and since people’s attention is limited, influence operations can be, in effect, a form of censorship by making authentic voices and opinions less visible.
References
- ^ have been widespread (www.brennancenter.org)
- ^ Russia (apnews.com)
- ^ China (www.reuters.com)
- ^ Iran (www.politico.com)
- ^ Israel (www.npr.org)
- ^ social bots (www.npr.org)
- ^ influencers (apnews.com)
- ^ media companies (www.reuters.com)
- ^ generative AI (www.forbes.com)
- ^ Observatory on Social Media (osome.iu.edu)
- ^ and I (scholar.google.com)
- ^ to detect (doi.org)
- ^ State-of-the-art methods (doi.org)
- ^ suspiciously similar sequences of actions (doi.org)
- ^ deleted to evade detection (doi.org)
- ^ AI-generated faces (doi.org)
- ^ dramatically cut the platform’s trust and safety teams (apnews.com)
- ^ bots that used ChatGPT (doi.org)
- ^ unable to distinguish (doi.org)
- ^ collecting (independenttechresearch.org)
- ^ data (independenttechresearch.org)
- ^ ethical experiments (www.science.org)
- ^ sway election outcomes (www.nbcnews.com)
- ^ simulates how information spreads through the social network (doi.org)
- ^ more – not less – content moderation (theconversation.com)
- ^ add friction (doi.org)
- ^ nudging users to reshare accurate information (doi.org)
- ^ AI content dissemination (doi.org)
- ^ protect, rather than censor, free speech (doi.org)
Authors: Filippo Menczer, Professor of Informatics and Computer Science, Indiana University