3 ways that big data reveals what you really like to watch, read and listen to
- Written by Anjana Susarla, Associate Professor of Information Systems, Michigan State University
Anyone who’s watched “Bridget Jones’s Diary” knows one of her New Year’s resolutions is “Not go out every night but stay in and read books and listen to classical music.”
The reality, however, is substantially different. What people actually do in their leisure time often doesn’t match with what they say they’ll do.
Economists have termed this phenomenon “hyperbolic discounting.” In a famous study titled “Paying Not to Go to the Gym[1],” a couple of economists found that, when people were offered the choice between a pay-per-visit contract and a monthly fee, they were more likely to choose the monthly fee and actually ended up paying more per visit. That’s because they overestimated their motivation to work out.
Hyperbolic discounting is just one challenge of operating in a creative industry. Tastes are highly subjective, and the elements of plot and narrative that make one movie a tremendous hit could easily make another a critical and commercial failure.
For decades, advertisers and marketers struggled to predict the consumption of leisure products such as movies and books. It’s equally challenging to decide the timing. Which weekend should a studio release a new movie? When a publisher releases a hard copy of a book, how do they decide when to release the e-book version?
Today, big data offers new visibility into how people experience entertainment. As a researcher who studies[2] the impact of artificial intelligence and social media, there are three forces that stand out to me as especially powerful in predicting human behavior.
1. Economics of the long tail
The internet makes it possible to distribute entertainment products that are less popular than mainstream successes. Streaming shows can acquire a larger audience than what is economically feasible for distribution through prime-time television. This economic phenomenon is referred to as the long tail effect[3],
Since streaming media companies such as Netflix do not have to pay to distribute content in movie theaters, they can produce more shows that cater to niche audiences. Netflix used data from their individual customers’ viewing habits to decide to back “House of Cards,” which was rejected by television networks[4]. Netflix data showed that there was a fan base for movies directed by Fincher and movies starring Spacey, and that a large number of customers had rented DVDs of the original BBC series.
2. Social influence in the era of artificial intelligence
With social media, people can share what they are watching with their friends, making otherwise independent entertainment experiences become more social.
By mining data from social sites like Twitter and Instagram, companies can track in real time what moviegoers think about a given movie, show or song. Movie studios can use a treasure trove of digital data to decide how to promote shows and release dates for movies. For instance, the volume of Google searches of a film’s trailer during the month before its premiere[5] is a leading predictor of Oscar winners as well as box office revenue. Movie studios can combine historical data about movie release dates and box office performance with search trends[6] to predict ideal release dates for new movies[7].
Mining social media data also helps companies to identify negative sentiment before it spirals into a crisis. A single tweet from an unhappy influential customer[8] can go viral, shaping public opinion.
In a study I conducted[9] with Yong Tan of the University of Washington and Cath Oh from Georgia State University, we showed[10] how such social influence determines not only which YouTube videos become more popular, but also that videos shared by influential users become even more widely viewed.
One study[11] shows that when studios pay attention to social media buzz before a movie’s release, the difference between the predicted revenue and the actual revenue, known as the forecast error, reduced by 31 percent.
3. Consumption analytics
Big data provides better visibility into what books and shows people actually spend their time enjoying.
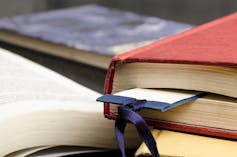
The mathematician Jordan Ellenberg pioneered the use of the Hawking index[13], a measure of the average page number of the five most highlighted passages in a Kindle book as a proportion of that book’s total length. The Hawking index shows when people give up on a book. If a 250-page book’s average Kindle highlight appears on page 250, that would give it a Hawking index of 100 percent.
The theory gets its name from Stephen Hawking’s “A Brief History in Time.” While this book still sells millions of copies a year, it is also seldom read, with a dismal Hawking index of 6.6 percent.
When a company such as Amazon decides which books to recommend to potential readers or which Prime shows to produce, they look at detailed digital traces of which plot points engaged audiences and which did not[14]. This might help them to promote an upcoming release or to make better recommendations to individual users.
What’s more, new types of artificial intelligence can investigate what makes people engage with creative content. For instance, a company named Epagogix pioneered an approach using a neural network – an artificial intelligence tool[15] that looks for patterns in very large amounts of data – on a set of screenplays rated by experts in the entertainment industry. The computer could then predict the financial success of a movie. According to some reports, such artificial intelligence can predict[16] up to 75 percent of films’ actual opening grosses.
Given new big data insights like these, entertainment companies may soon know what exactly Bridget Jones would like to do with her leisure time better than Bridget herself does.
References
- ^ Paying Not to Go to the Gym (doi.org)
- ^ researcher who studies (scholar.google.com)
- ^ long tail effect (www.hachettebooks.com)
- ^ was rejected by television networks (mitpress.mit.edu)
- ^ Google searches of a film’s trailer during the month before its premiere (www.theverge.com)
- ^ search trends (doi.org)
- ^ predict ideal release dates for new movies (datavisitor.com)
- ^ A single tweet from an unhappy influential customer (theconversation.com)
- ^ In a study I conducted (doi.org)
- ^ we showed (theconversation.com)
- ^ One study (doi.org)
- ^ diego matteo muzzini/shutterstock.com (www.shutterstock.com)
- ^ Hawking index (www.wsj.com)
- ^ which plot points engaged audiences and which did not (www.thrillist.com)
- ^ an artificial intelligence tool (www.newyorker.com)
- ^ such artificial intelligence can predict (fortune.com)
Authors: Anjana Susarla, Associate Professor of Information Systems, Michigan State University