Generative AI could leave users holding the bag for copyright violations
- Written by Anjana Susarla, Professor of Information Systems, Michigan State University
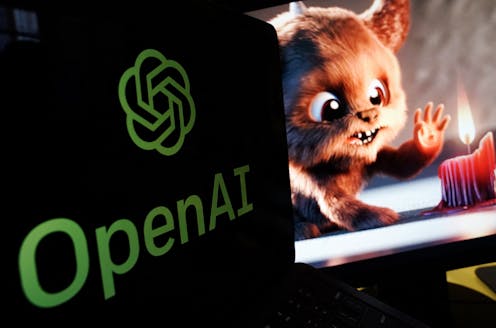
Generative artificial intelligence has been hailed for its potential to transform creativity[1], and especially by lowering the barriers to content creation[2]. While the creative potential of generative AI tools[3] has often been highlighted, the popularity of these tools poses questions about intellectual property and copyright protection.
Generative AI tools such as ChatGPT are powered by foundational AI models[4], or AI models trained on vast quantities of data[5]. Generative AI is trained on[6] billions of pieces of data taken from text or images scraped from the internet.
Generative AI uses very powerful machine learning methods such as deep learning[7] and transfer learning[8] on such vast repositories of data to understand the relationships among those pieces of data – for instance, which words tend to follow other words. This allows generative AI to perform a broad range of tasks that can mimic cognition and reasoning[9].
One problem is that output from an AI tool can be very similar to copyright-protected materials[10]. Leaving aside how generative models are trained, the challenge that widespread use of generative AI poses is how individuals and companies could be held liable when generative AI outputs infringe on copyright protections.
When prompts result in copyright violations
Researchers[11] and journalists[12] have raised the possibility that through selective prompting strategies, people can end up creating text, images or video that violates copyright law. Typically, generative AI tools output an image, text or video but do not provide any warning about potential infringement[13]. This raises the question of how to ensure that users of generative AI tools do not unknowingly end up infringing copyright protection.
The legal argument advanced by generative AI companies is that AI trained on copyrighted works is not an infringement of copyright since these models are not copying the training data[14]; rather, they are designed to learn the associations between the elements of writings and images like words and pixels. AI companies, including Stability AI, maker of image generator Stable Diffusion, contend that output images provided in response to a particular text prompt is not likely to be a close match[15] for any specific image in the training data.
Builders of generative AI tools have argued that prompts do not reproduce the training data, which should protect them from claims of copyright violation. Some audit studies have shown, though, that end users of generative AI[17] can issue prompts that result in copyright violations[18] by producing works that closely resemble copyright-protected content[19].
Establishing infringement requires detecting a close resemblance[20] between expressive elements of a stylistically similar work and original expression in particular works by that artist. Researchers have shown that methods such as training data extraction attacks[21], which involve selective prompting strategies, and extractable memorization[22], which tricks generative AI systems into revealing training data, can recover individual training examples ranging from photographs of individuals to trademarked company logos.
Audit studies such as the one conducted by computer scientist Gary Marcus and artist Reid Southern[23] provide several examples where there can be little ambiguity about the degree to which visual generative AI models produce images that infringe on copyright protection. The New York Times provided a similar comparison of images showing how generative AI tools can violate copyright protection[24].
How to build guardrails
Legal scholars have dubbed the challenge in developing guardrails against copyright infringement into AI tools the “Snoopy problem[25].” The more a copyrighted work is protecting a likeness – for example, the cartoon character Snoopy – the more likely it is a generative AI tool will copy it compared to copying a specific image.
Researchers in computer vision have long grappled with the issue[26] of how to detect copyright infringement, such as logos that are counterfeited or images that are protected by patents[27]. Researchers have also examined how logo detection can help identify counterfeit products[28]. These methods can be helpful in detecting violations of copyright. Methods to establish content provenance and authenticity[29] could be helpful as well.
With respect to model training, AI researchers have suggested methods for making generative AI models unlearn[30] copyrighted data[31]. Some AI companies such as Anthropic have announced pledges[32] to not use data produced by their customers to train advanced models such as Anthropic’s large language model Claude. Methods for AI safety such as red teaming[33] – attempts to force AI tools to misbehave – or ensuring that the model training process reduces the similarity[34] between the outputs of generative AI and copyrighted material may help as well.
Artists and technologists are fighting back against AI copyright infringement.Role for regulation
Human creators know to decline requests to produce content that violates copyright. Can AI companies build similar guardrails into generative AI?
There’s no established approaches to build such guardrails into generative AI, nor are there any public tools or databases that users can consult[35] to establish copyright infringement. Even if tools like these were available, they could put an excessive burden on both users and content providers[36].
Given that naive users can’t be expected to learn and follow best practices to avoid infringing copyrighted material, there are roles for policymakers and regulation. It may take a combination of legal and regulatory guidelines to ensure best practices for copyright safety.
For example, companies that build generative AI models could use filtering or restrict model outputs[37] to limit copyright infringement. Similarly, regulatory intervention may be necessary to ensure that builders of generative AI models build datasets and train models[38] in ways that reduce the risk that the output of their products infringe creators’ copyrights.
References
- ^ potential to transform creativity (doi.org)
- ^ barriers to content creation (doi.org)
- ^ creative potential of generative AI tools (doi.org)
- ^ foundational AI models (research.ibm.com)
- ^ trained on vast quantities of data (hai.stanford.edu)
- ^ trained on (news.mit.edu)
- ^ deep learning (doi.org)
- ^ transfer learning (www.datacamp.com)
- ^ mimic cognition and reasoning (doi.org)
- ^ very similar to copyright-protected materials (crsreports.congress.gov)
- ^ Researchers (doi.org)
- ^ journalists (www.tomshardware.com)
- ^ do not provide any warning about potential infringement (spectrum.ieee.org)
- ^ since these models are not copying the training data (doi.org)
- ^ is not likely to be a close match (www.hollywoodreporter.com)
- ^ AP Photo/George Walker IV (newsroom.ap.org)
- ^ end users of generative AI (www.tomshardware.com)
- ^ prompts that result in copyright violations (spectrum.ieee.org)
- ^ closely resemble copyright-protected content (garymarcus.substack.com)
- ^ detecting a close resemblance (houstonlawreview.org)
- ^ training data extraction attacks (dx.doi.org)
- ^ extractable memorization (doi.org)
- ^ conducted by computer scientist Gary Marcus and artist Reid Southern (spectrum.ieee.org)
- ^ can violate copyright protection (www.nytimes.com)
- ^ the “Snoopy problem (dx.doi.org)
- ^ have long grappled with the issue (doi.org)
- ^ images that are protected by patents (doi.org)
- ^ logo detection can help identify counterfeit products (doi.org)
- ^ establish content provenance and authenticity (doi.org)
- ^ generative AI models unlearn (doi.org)
- ^ copyrighted data (openaccess.thecvf.com)
- ^ Anthropic have announced pledges (claudeai.uk)
- ^ red teaming (doi.org)
- ^ reduces the similarity (doi.org)
- ^ public tools or databases that users can consult (spectrum.ieee.org)
- ^ both users and content providers (spectrum.ieee.org)
- ^ use filtering or restrict model outputs (dx.doi.org)
- ^ build datasets and train models (dx.doi.org)
Authors: Anjana Susarla, Professor of Information Systems, Michigan State University