AI and new standards promise to make scientific data more useful by making it reusable and accessible
- Written by Bradley Wade Bishop, Professor of Information Sciences, University of Tennessee
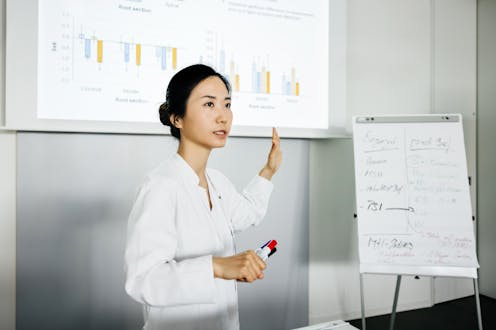
Every time a scientist runs an experiment, or a social scientist does a survey, or a humanities scholar analyzes a text, they generate data. Science runs on data – without it, we wouldn’t have the James Webb Space Telescope’s stunning images[1], disease-preventing vaccines[2] or an evolutionary tree that traces the lineages[3] of all life.
This scholarship generates an unimaginable amount of data – so how do researchers keep track of it? And how do they make sure that it’s accessible for use by both humans and machines?
To improve and advance science, scientists need to be able to reproduce[4] others’ data or combine data from multiple sources to learn something new.
Accessible and usable data can help scientists reproduce prior results. Doing so is an important part of the scientific process, as this TED-Ed video explains.Any kind of sharing requires management. If your neighbor needs to borrow a tool or an ingredient, you have to know whether you have it and where you keep it. Research data might be on a graduate student’s laptop, buried in a professor’s USB collection or saved more permanently within an online data repository.
I’m an information scientist[5] who studies other scientists. More precisely, I study how scientists think about research data and the ways that they interact with their own data and data from others. I also teach students how to manage their own or others’ data in ways that advance knowledge.
Research data management
Research data management[6] is an area of scholarship that focuses on data discovery and reuse. As a field, it encompasses research data services, resources and cyberinfrastructure. For example, one type of infrastructure, the data repository[7], gives researchers a place to deposit their data for long-term storage so that others can find it. In short, research data management encompasses the data’s life cycle from cradle to grave to reincarnation in the next study.
Proper research data management also allows scientists to use the data already out there rather than recollecting data that already exists, which saves time and resources.
With increasing science politicization[8], many national and international science organizations have upped their standards for accountability and transparency[9]. Federal agencies[10] and other major research funders like the National Institutes of Health[11] now prioritize research data management and require researchers to have a data management plan before they can receive any funds.
Scientists and data managers can work together to redesign the systems scientists use to make data discovery and preservation easier. In particular, integrating AI[12] can make this data more accessible and reusable.
Artificially intelligent data management
Many of these new standards for research data management also stem from an increased use of AI, including machine learning, across data-driven fields[13]. AI makes it highly desirable for any data to be machine-actionable – that is, usable by machines without human intervention. Now, scholars can consider machines not only as tools but also as potential autonomous data reusers and collaborators.
The key to machine-actionable data is metadata. Metadata[14] are the descriptions scientists set for their data and may include elements such as creator, date, coverage and subject. Minimal metadata is minimally useful, but correct and complete standardized metadata makes data more useful for both people and machines.
It takes a cadre of research data managers and librarians to make machine-actionable data a reality. These information professionals[15] work to facilitate communication between scientists and systems by ensuring the quality, completeness and consistency of shared data.
The FAIR data principles[16], created by a group of researchers called FORCE11[17] in 2016 and used across the world, provide guidance on how to enable data reuse by machines and humans. FAIR data is findable, accessible, interoperable and reusable – meaning it has robust and complete metadata.
In the past, I’ve studied how scientists discover and reuse data[18]. I found that scientists tend to use mental shortcuts when they’re looking for data – for example, they may go back to familiar and trusted sources or search for certain key terms they’ve used before. Ideally, my team could build this decision-making process of experts and remove as many biases as possible to improve AI. The automation of these mental shortcuts should reduce the time-consuming chore of locating the right data.
Data management plans
But there’s still one piece of research data management that AI can’t take over. Data management plans[20] describe the what, where, when, why and who of managing research data. Scientists fill them out, and they outline the roles and activities for managing research data during and long after research ends. They answer questions like, “Who is responsible for long-term preservation,” “Where will the data live,” “How do I keep my data secure,” and “Who pays for all of that?”
Grant proposals for nearly all funding agencies across countries now require data management plans[21]. These plans signal to scientists that their data is valuable and important enough to the community to share. Also, the plans help funding agencies keep tabs on the research and investigate any potential misconduct[22]. But most importantly, they help scientists make sure their data stays accessible for many years.
Making all research data as FAIR and open as possible will improve the scientific process. And having access to more data opens up the possibility for more informed discussions on how to promote[23] economic development, improve the stewardship of natural resources, enhance public health, and how to responsibly and ethically develop technologies that will improve lives. All intelligence, artificial or otherwise, will benefit from better organization, access and use of research data.
References
- ^ stunning images (webbtelescope.org)
- ^ vaccines (www.cdc.gov)
- ^ traces the lineages (www.gbif.org)
- ^ be able to reproduce (www.nature.com)
- ^ information scientist (bradleywadebishop.github.io)
- ^ Research data management (www.oclc.org)
- ^ data repository (www.re3data.org)
- ^ increasing science politicization (doi.org)
- ^ standards for accountability and transparency (nap.nationalacademies.org)
- ^ Federal agencies (www.whitehouse.gov)
- ^ National Institutes of Health (sharing.nih.gov)
- ^ integrating AI (doi.org)
- ^ data-driven fields (doi.org)
- ^ Metadata (www.dublincore.org)
- ^ information professionals (doi.org)
- ^ FAIR data principles (www.go-fair.org)
- ^ FORCE11 (force11.org)
- ^ how scientists discover and reuse data (doi.org)
- ^ Penpak Ngamsathain/Moment via Getty Images (www.gettyimages.com)
- ^ Data management plans (doi.org)
- ^ now require data management plans (theconversation.com)
- ^ investigate any potential misconduct (doi.org)
- ^ how to promote (www.fgdc.gov)
Authors: Bradley Wade Bishop, Professor of Information Sciences, University of Tennessee