AI can help forecast air quality, but freak events like 2023's summer of wildfire smoke require traditional methods too
- Written by Joshua S. Fu, Chancellor's Professor in Engineering, Climate Change and Civil and Environmental Engineering, University of Tennessee
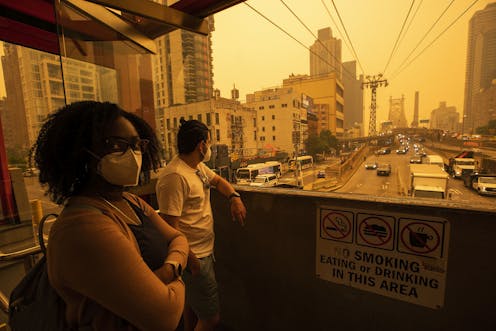
Wildfire smoke from Canada’s extreme fire season[1] has left a lot of people thinking about air quality and wondering what to expect in the days ahead.
All air contains gaseous compounds and small particles. But as air quality gets worse, these gases and particles can trigger asthma[2] and exacerbate heart and respiratory problems[3] as they enter the nose, throat and lungs and even circulate in the bloodstream. When wildfire smoke turned New York City’s skies orange in early June 2023, emergency room visits[4] for asthma doubled.
In most cities[5], it’s easy to find a daily air quality index score[6] that tells you when the air is considered unhealthy or even hazardous. However, predicting air quality in the days ahead isn’t so simple.
I work on air quality forecasting as a professor of civil and environmental engineering[7]. Artificial intelligence has improved these forecasts, but research shows it’s much more useful when paired with traditional techniques. Here’s why:
How scientists predict air quality
To predict air quality in the near future – a few days ahead or longer – scientists generally rely on two main methods[8]: a chemical transport model[9] or a machine-learning model. These two models generate results in totally different ways.
Chemical transport models use lots of known chemical and physical formulas to calculate the presence and production of air pollutants. They use data from emissions inventories reported by local agencies that list pollutants from known sources, such as wildfires, traffic or factories[10], and data from meteorology that provides atmospheric information, such as wind, precipitation, temperature and solar radiation.
These models simulate the flow and chemical reactions of the air pollutants. However, their simulations involve multiple variables with huge uncertainties. Cloudiness, for example, changes the incoming solar radiation and thus the photochemistry. This can make the results less accurate.
Machine-learning models instead learn patterns over time from historical data to predict future air quality for any given region, and then apply that knowledge to current conditions to predict the future.
The downside of machine-learning models is that they do not consider any chemical and physical mechanisms, as chemical transport models do. Also, the accuracy of machine-learning projections under extreme conditions, such as heat waves or wildfire events, can be off if the models weren’t trained on such data. So, while machine-learning models can show where and when high pollution levels are most likely, such as during rush hour near freeways, they generally cannot deal with more random events, like wildfire smoke blowing in from Canada.
Which is better?
Scientists have determined that neither model is accurate enough on its own, but using the best attributes of both[12] models together can help better predict the quality[13] of the air we breathe.
This combined method, known as the machine-learning – measurement model fusion, or ML-MMF, has the ability to provide science-based predictions with more than 90% accuracy[14]. It is based on known physical and chemical mechanisms and can simulate the whole process, from the air pollution source to your nose. Adding satellite data can help them inform the public on both air quality safety levels and the direction pollutants are traveling with greater accuracy.
We recently compared predictions from all three models[15] with actual pollution measurements. The results were striking: The combined model was 66% more accurate than the chemical transport model and 12% more accurate than the machine-learning model alone.
The chemical transport model is still the most common method used today to predict air quality, but applications with machine-learning models are becoming more popular. The regular forecasting method[16] used by the U.S. Environmental Protection Agency’s AirNow.gov[17] relies on machine learning. The site also compiles air quality forecast results from state and local agencies, most of which use chemical transport[18] models[19].
As information sources become more reliable, the combined models will become more accurate ways to forecast hazardous air quality, particularly during unpredictable events like wildfire smoke.
References
- ^ Canada’s extreme fire season (twitter.com)
- ^ trigger asthma (theconversation.com)
- ^ exacerbate heart and respiratory problems (theconversation.com)
- ^ emergency room visits (gothamist.com)
- ^ most cities (www.airnow.gov)
- ^ air quality index score (www.lung.org)
- ^ professor of civil and environmental engineering (cee.utk.edu)
- ^ main methods (www.airnow.gov)
- ^ chemical transport model (www.airnow.gov)
- ^ or factories (www.epa.gov)
- ^ AirNow.gov (gispub.epa.gov)
- ^ best attributes of both (doi.org)
- ^ can help better predict the quality (doi.org)
- ^ more than 90% accuracy (doi.org)
- ^ compared predictions from all three models (doi.org)
- ^ forecasting method (gispub.epa.gov)
- ^ AirNow.gov (www.airnow.gov)
- ^ chemical transport (www.epa.gov)
- ^ models (www.camx.com)
Authors: Joshua S. Fu, Chancellor's Professor in Engineering, Climate Change and Civil and Environmental Engineering, University of Tennessee