Cross-pollination among neuroscience, psychology and AI research yields a foundational understanding of thinking
- Written by Paul S. Rosenbloom, Professor Emeritus of Computer Science, University of Southern California
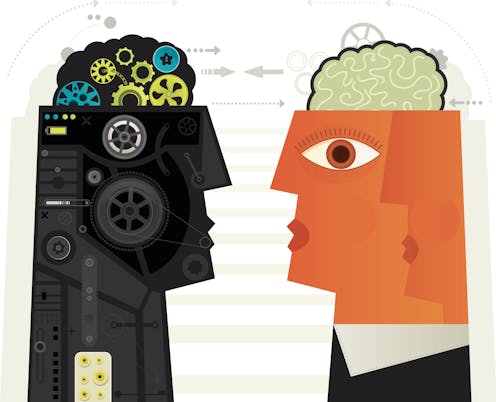
Progress in artificial intelligence[1] has enabled the creation of AIs that perform tasks previously thought only possible for humans, such as translating languages[2], driving cars[3], playing board games at world-champion level[4] and extracting the structure of proteins[5]. However, each of these AIs has been designed and exhaustively trained for a single task and has the ability to learn only what’s needed for that specific task.
Recent AIs that produce fluent text[6], including in conversation with humans, and generate impressive and unique art[7] can give the false impression of a mind at work[8]. But even these are specialized systems that carry out narrowly defined tasks and require massive amounts of training.
It still remains a daunting challenge to combine multiple AIs into one that can learn and perform many different tasks, much less pursue the full breadth of tasks performed by humans or leverage the range of experiences available to humans that reduce the amount of data otherwise required to learn how to perform these tasks. The best current AIs in this respect, such as AlphaZero[9] and Gato[10], can handle a variety of tasks that fit a single mold, like game-playing. Artificial general intelligence (AGI)[11] that is capable of a breadth of tasks remains elusive.
Ultimately, AGIs need to be able to[12] interact effectively with each other and people in various physical environments and social contexts, integrate the wide varieties of skill and knowledge needed to do so, and learn flexibly and efficiently from these interactions.
Building AGIs comes down to building artificial minds, albeit greatly simplified compared to human minds. And to build an artificial mind, you need to start with a model of cognition.
From human to Artificial General Intelligence
Humans have an almost unbounded set of skills and knowledge, and quickly learn new information without needing to be re-engineered to do so. It is conceivable that an AGI can be built using an approach that is fundamentally different from human intelligence. However, as three longtime researchers[14] in AI and cognitive science[15], our approach is to draw inspiration and insights from the structure of the human mind. We are working toward AGI by trying to better understand the human mind, and better understand the human mind by working toward AGI.
From research in neuroscience, cognitive science and psychology, we know that the human brain is neither a huge homogeneous set of neurons nor a massive set of task-specific programs that each solves a single problem. Instead, it is a set of regions with different properties[16] that support the basic cognitive capabilities that together form the human mind.
These capabilities include perception and action; short-term memory for what is relevant in the current situation; long-term memories for skills, experience and knowledge; reasoning and decision making; emotion and motivation; and learning new skills and knowledge from the full range of what a person perceives and experiences.
Instead of focusing on specific capabilities in isolation, AI pioneer Allen Newell[17] in 1990 suggested developing Unified Theories of Cognition[18] that integrate all aspects of human thought. Researchers have been able to build software programs called cognitive architectures[19] that embody such theories, making it possible to test and refine them.
Cognitive architectures are grounded in multiple scientific fields with distinct perspectives. Neuroscience focuses on the organization of the human brain, cognitive psychology on human behavior in controlled experiments, and artificial intelligence on useful capabilities.
The Common Model of Cognition
We have been involved in the development of three cognitive architectures: ACT-R[20], Soar[21] and Sigma[22]. Other researchers have also been busy on alternative approaches. One paper identified nearly 50 active cognitive architectures[23]. This proliferation of architectures is partly a direct reflection of the multiple perspectives involved, and partly an exploration of a wide array of potential solutions. Yet, whatever the cause, it raises awkward questions both scientifically and with respect to finding a coherent path to AGI.
Fortunately, this proliferation has brought the field to a major inflection point. The three of us have identified a striking convergence among architectures, reflecting a combination of neural, behavioral and computational studies. In response, we initiated a communitywide effort to capture this convergence[24] in a manner akin to the Standard Model of Particle Physics[25] that emerged in the second half of the 20th century.
This Common Model of Cognition[27] divides humanlike thought into multiple modules, with a short-term memory module at the center of the model. The other modules – perception, action, skills and knowledge – interact through it.
Learning, rather than occurring intentionally, happens automatically as a side effect of processing. In other words, you don’t decide what is stored in long-term memory. Instead, the architecture determines what is learned based on whatever you do think about. This can yield learning of new facts you are exposed to or new skills that you attempt. It can also yield refinements to existing facts and skills.
The modules themselves operate in parallel; for example, allowing you to remember something while listening and looking around your environment. Each module’s computations are massively parallel, meaning many small computational steps happening at the same time. For example, in retrieving a relevant fact from a vast trove of prior experiences, the long-term memory module can determine the relevance of all known facts simultaneously, in a single step.
Guiding the way to Artificial General Intelligence
The Common Model is based on the current consensus in research in cognitive architectures and has the potential to guide research on both natural and artificial general intelligence. When used to model communication patterns in the brain, the Common Model yields more accurate results than leading models from neuroscience. This extends its ability to model humans[28] – the one system proven capable of general intelligence – beyond cognitive considerations to include the organization of the brain itself.
We are starting to see efforts to relate existing cognitive architectures to the Common Model and to use it as a baseline for new work – for example, an interactive AI designed to coach people[29] toward better health behavior. One of us was involved in developing an AI based on Soar, dubbed Rosie[30], that learns new tasks via instructions in English from human teachers. It learns 60 different puzzles and games and can transfer what it learns from one game to another. It also learns to control a mobile robot for tasks such as fetching and delivering packages and patrolling buildings.
Rosie is just one example of how to build an AI that approaches AGI via a cognitive architecture that is well characterized by the Common Model. In this case, the AI automatically learns new skills and knowledge during general reasoning that combines natural language instruction from humans and a minimal amount of experience – in other words, an AI that functions more like a human mind than today’s AIs, which learn via brute computing force and massive amounts of data.
From a broader AGI perspective, we look to the Common Model both as a guide in developing such architectures and AIs, and as a means for integrating the insights derived from those attempts into a consensus that ultimately leads to AGI.
References
- ^ artificial intelligence (www.aaai.org)
- ^ translating languages (aclanthology.org)
- ^ driving cars (www.nhtsa.gov)
- ^ playing board games at world-champion level (deepmind.com)
- ^ extracting the structure of proteins (doi.org)
- ^ fluent text (theconversation.com)
- ^ generate impressive and unique art (theconversation.com)
- ^ false impression of a mind at work (theconversation.com)
- ^ AlphaZero (doi.org)
- ^ Gato (www.deepmind.com)
- ^ Artificial general intelligence (AGI) (www.agi-society.org)
- ^ need to be able to (doi.org)
- ^ CC BY-ND (creativecommons.org)
- ^ researchers (scholar.google.com)
- ^ cognitive science (scholar.google.com)
- ^ set of regions with different properties (www.hopkinsmedicine.org)
- ^ Allen Newell (www.computer.org)
- ^ Unified Theories of Cognition (www.hup.harvard.edu)
- ^ cognitive architectures (doi.org)
- ^ ACT-R (act-r.psy.cmu.edu)
- ^ Soar (soar.eecs.umich.edu)
- ^ Sigma (cogarch.ict.usc.edu)
- ^ identified nearly 50 active cognitive architectures (doi.org)
- ^ a communitywide effort to capture this convergence (ojs.library.carleton.ca)
- ^ Standard Model of Particle Physics (home.cern)
- ^ CC BY-ND (creativecommons.org)
- ^ Common Model of Cognition (doi.org)
- ^ extends its ability to model humans (doi.org)
- ^ designed to coach people (doi.org)
- ^ Rosie (soargroup.github.io)
Authors: Paul S. Rosenbloom, Professor Emeritus of Computer Science, University of Southern California