AI and machine learning are improving weather forecasts, but they won't replace human experts
- Written by Russ Schumacher, Associate Professor of Atmospheric Science and Colorado State Climatologist, Colorado State University
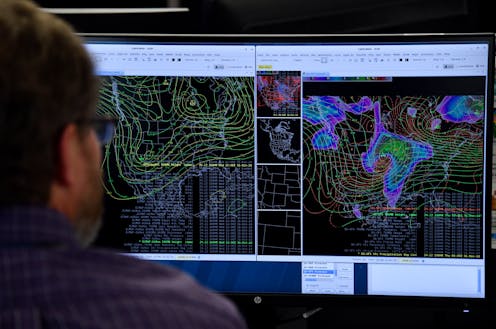
A century ago, English mathematician Lewis Fry Richardson[1] proposed a startling idea for that time: constructing a systematic process based on math for predicting the weather. In his 1922 book, “Weather Prediction By Numerical Process[2],” Richardson tried to write an equation that he could use to solve the dynamics of the atmosphere based on hand calculations.
It didn’t work because not enough was known about the science of the atmosphere at that time. “Perhaps some day in the dim future it will be possible to advance the computations faster than the weather advances and at a cost less than the saving to mankind due to the information gained. But that is a dream,” Richardson concluded.
A century later, modern weather forecasts are based on the kind of complex computations that Richardson imagined[3] – and they’ve become more accurate than anything he envisioned. Especially in recent decades, steady progress in research, data and computing has enabled a “quiet revolution of numerical weather prediction[4].”
For example, a forecast of heavy rainfall two days in advance is now as good[5] as a same-day forecast was in the mid-1990s. Errors in the predicted tracks of hurricanes have been cut in half[6] in the last 30 years.
There still are major challenges. Thunderstorms that produce tornadoes, large hail or heavy rain remain difficult to predict. And then there’s chaos, often described as the “butterfly effect” – the fact that small changes in complex processes make weather less predictable[7]. Chaos limits our ability to make precise forecasts beyond about 10 days[8].
As in many other scientific fields, the proliferation of tools like artificial intelligence and machine learning holds great promise for weather prediction. We have seen some of what’s possible in our research[9] on applying machine learning[10] to forecasts of high-impact weather. But we also believe that while these tools open up new possibilities for better forecasts, many parts of the job are handled more skillfully by experienced people.
Australian meteorologist Dean Narramore explains why it’s hard to forecast large thunderstorms.Predictions based on storm history
Today, weather forecasters’ primary tools are numerical weather prediction models[11]. These models use observations of the current state of the atmosphere from sources such as weather stations, weather balloons and satellites, and solve equations that govern the motion of air.
These models are outstanding at predicting most weather systems, but the smaller a weather event is, the more difficult it is to predict. As an example, think of a thunderstorm that dumps heavy rain on one side of town and nothing on the other side. Furthermore, experienced forecasters are remarkably good at synthesizing the huge amounts of weather information they have to consider each day, but their memories and bandwidth are not infinite.
Artificial intelligence and machine learning can help with some of these challenges. Forecasters are using these tools in several ways now, including making predictions of high-impact weather that the models can’t provide.
In a project that started in 2017 and was reported in a 2021 paper[12], we focused on heavy rainfall. Of course, part of the problem is defining “heavy”: Two inches of rain in New Orleans may mean something very different than in Phoenix. We accounted for this by using observations of unusually large rain accumulations for each location across the country, along with a history of forecasts from a numerical weather prediction model.
We plugged that information into a machine learning method known as “random forests[13],” which uses many decision trees to split a mass of data and predict the likelihood of different outcomes. The result is a tool that forecasts the probability that rains heavy enough to generate flash flooding will occur.
We have since applied similar methods to forecasting of tornadoes, large hail and severe thunderstorm winds. Other[14] research[15] groups[16] are developing similar tools. National Weather Service forecasters are using some of these tools to better assess the likelihood of hazardous weather on a given day.
Researchers also are embedding machine learning within numerical weather prediction models to speed up tasks that can be intensive to compute, such as predicting how water vapor gets converted to rain, snow or hail.
It’s possible that machine learning models could eventually replace traditional numerical weather prediction models altogether. Instead of solving a set of complex physical equations as the models do, these systems instead would process thousands of past weather maps to learn how weather systems tend to behave. Then, using current weather data, they would make weather predictions based on what they’ve learned from the past.
Some studies have shown that machine learning-based forecast systems can predict general weather patterns[18] as well as numerical weather prediction models[19] while using only a fraction of the computing power the models require. These new tools don’t yet forecast the details of local weather that people care about, but with many researchers carefully testing them and inventing new methods, there is promise for the future.
The role of human expertise
There are also reasons for caution. Unlike numerical weather prediction models, forecast systems that use machine learning are not constrained by the physical laws that govern the atmosphere. So it’s possible that they could produce unrealistic results – for example, forecasting temperature extremes beyond the bounds of nature. And it is unclear how they will perform during highly unusual or unprecedented weather phenomena.
And relying on AI tools can raise ethical concerns[21]. For instance, locations with relatively few weather observations with which to train a machine learning system may not benefit from forecast improvements that are seen in other areas.
Another central question is how best to incorporate these new advances into forecasting. Finding the right balance between automated tools and the knowledge of expert human forecasters has long been a challenge in meteorology. Rapid technological advances will only make it more complicated.
Ideally, AI and machine learning will allow human forecasters to do their jobs more efficiently, spending less time on generating routine forecasts and more on communicating forecasts’ implications and impacts to the public – or, for private forecasters, to their clients. We believe that careful[22] collaboration[23] between scientists, forecasters and forecast users is the best way to achieve these goals and build trust in machine-generated weather forecasts.
References
- ^ Lewis Fry Richardson (mathshistory.st-andrews.ac.uk)
- ^ Weather Prediction By Numerical Process (www.emetsoc.org)
- ^ computations that Richardson imagined (www.irishtimes.com)
- ^ quiet revolution of numerical weather prediction (doi.org)
- ^ now as good (www.wpc.ncep.noaa.gov)
- ^ cut in half (www.nhc.noaa.gov)
- ^ less predictable (www.discovery.com)
- ^ beyond about 10 days (doi.org)
- ^ our research (scholar.google.com)
- ^ applying machine learning (scholar.google.com)
- ^ numerical weather prediction models (www.ncei.noaa.gov)
- ^ 2021 paper (doi.org)
- ^ random forests (www.ibm.com)
- ^ Other (doi.org)
- ^ research (www2.mmm.ucar.edu)
- ^ groups (doi.org)
- ^ CC BY-ND (creativecommons.org)
- ^ can predict general weather patterns (doi.org)
- ^ numerical weather prediction models (ai.googleblog.com)
- ^ CC BY-ND (creativecommons.org)
- ^ ethical concerns (doi.org)
- ^ careful (www.ai2es.org)
- ^ collaboration (www.tacc.utexas.edu)
Authors: Russ Schumacher, Associate Professor of Atmospheric Science and Colorado State Climatologist, Colorado State University