Including race in clinical algorithms can both reduce and increase health inequities – it depends on what doctors use them for
- Written by Anirban Basu, Professor of Health Economics, University of Washington
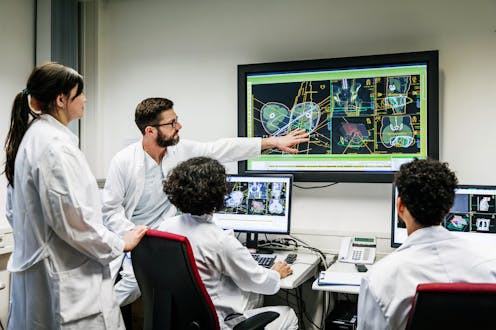
Health practitioners are increasingly concerned[1] that because race is a social construct, and the biological mechanisms of how race affects clinical outcomes are often unknown, including race in predictive algorithms for clinical decision-making may worsen inequities.
For example, to calculate an estimate of kidney function called the estimated glomerular filtration rate, or eGFR, health care providers use an algorithm based on age, biological sex, race (Black or non-Black) and serum creatinine, a waste product the kidneys release into the blood. A higher eGFR value means better kidney health. These eGFR predictions are used to allocate kidney transplants in the U.S.[2][3]
Based on this algorithm, which was trained on actual GFR values from patients[4], a Black patient would be assigned a higher eGFR than a non-Black patient of the same age, sex and serum creatinine level. This implies that some Black patients would be considered to have healthier kidneys than otherwise similar non-Black patients and less likely to be assigned a kidney transplant.
Biased clinical algorithms can lead to inaccurate diagnoses and delayed treatment.In 2021, however, researchers found that excluding race in the original eGFR equations could lead to larger discrepancies[5] between estimated and actual GFR values for both Black and non-Black patients. They also found adding an additional biomarker called cystatin C can improve predictions. However, even with this biomarker, excluding race from the algorithm still led to elevated discrepanies across races.
I am a health economist and statistician[6] who studies how unobserved factors in data can result in biases that lead to inefficiencies, inequities and disparities in health care. My recently published research suggests that excluding race from certain diagnostic algorithms could worsen health inequities[7].
Different approaches to fairness
Researchers use different economic frameworks[8] to understand how society allocates resources. Two key frameworks are utilitarianism and equality of opportunity.
A purely utilitarian outlook[9] seeks to identify what features would get the most out of a positive outcome or reduce the harm from a negative one, ignoring who possesses those features. This approach allocates resources to those with the most opportunities to generate positive outcomes or mitigate negative ones.
A utilitarian approach would always include race and ethnicity to improve the prediction power and accuracy of algorithms, regardless of whether it’s fair. For example, utilitarian policies would aim to maximize overall survival among people seeking organ transplants. They would allocate organs to those who would survive the longest from transplantation, even if those who may not survive the longest due to circumstances outside their control and need the organs most would die sooner without the transplant.
Although utilitarian approaches do not take fairness into account, an approach that does would ask two questions: How do we define fairness? Are there conditions when maximizing an algorithm’s prediction power and accuracy would not conflict with fairness?
To answer these questions, I apply the equality of opportunity[10] framework, which aims to allocate resources in a way that allows everyone the same chance of obtaining similar outcomes, without being disadvantaged by circumstances outside of their control. Researchers have used this framework in many contexts, such as political science[11], economics[12] and law[13]. The U.S. Supreme Court has also applied equality of opportunity in several landmark rulings in education[14].
Equality of opportunity
There are two fundamental principles in equality of opportunity.
First, inequality of outcomes is unethical if it results from differences in circumstances that are outside of an individual’s own control, such as the income of a child’s parents, exposure to systemic racism or living in violent and unsafe environments[16]. This can be remedied by compensating individuals with disadvantaged circumstances in a way that allows them the same opportunity to obtain certain health outcomes as those who are not disadvantaged by their circumstances.
Second, inequality of outcomes for people in similar circumstances that result from differences in individual effort, such as practicing health-promoting behaviors like diet and exercise, is not unethical, and policymakers can reward those achieving better outcomes through such behaviors. However, differences in individual effort that occur because of circumstances, such as living in an area with limited access to healthy food[17], are not addressed under equality of opportunity. Keeping all circumstances the same, any differences in effort between individuals should be due to preferences, free will and perceived benefits and costs. This is called accountable effort[18]. So, two individuals with the same circumstances should be rewarded according to their accountable efforts, and society should accept the resulting differences in outcomes.
Equality of opportunity implies that if algorithms were to be used for clinical decision-making, then it is necessary to understand what causes variation in the predictions they make.
If variation in predictions results from differences in circumstances or biological conditions but not from individual accountable effort, then it is appropriate to use the algorithm for compensation, such as allocating kidneys so everyone has an equal opportunity to live the same length of life, but not for reward, such as allocating kidneys to those who would live the longest with the kidneys.
In contrast, if variation in predictions results from differences in individual accountable effort but not from their circumstances, then it is appropriate to use the algorithm for reward but not compensation.
Evaluating clinical algorithms for fairness
To hold machine learning and other artificial intelligence algorithms accountable to a standard of equity, I applied the principles of equality of opportunity to evaluate whether race should be included[19] in clinical algorithms. I ran simulations under both ideal data conditions, where all data on a person’s circumstances is available, and real data conditions, where some data on a person’s circumstances is missing.
In these simulations, I unequivocally assume that race is a social and not biological construct[20]. Variables such as race and ethnicity are often proxies for various circumstances[21] individuals face that are out of their control, such as systemic racism that contributes to health disparities.
As a social construct, race is often a proxy for nonbiological circumstances.I evaluated two categories of algorithms.
The first, diagnostic algorithms, makes predictions based on outcomes that have already occurred at the time of decision-making. For example, diagnostic algorithms are used to predict the presence of gallstones in patients with abdominal pain or urinary tract infections, or to detect breast cancer using radiologic imaging.
The second, prognostic algorithms, predicts future outcomes that have not yet occurred at the time of decision-making. For example, prognostic algorithms are used to predict whether a patient will live if they do or do not obtain a kidney transplant.
I found that, under an equality of opportunity approach, diagnostic models that do not take race into account would increase systemic inequities and discrimination[22]. I found similar results for prognostic models intended to compensate for individual circumstances. For example, excluding race from algorithms that predict the future survival of patients with kidney failure would fail to identify those with underlying circumstances that make them more vulnerable.
Including race in prognostic models intended to reward individual efforts can also increase disparities[23]. For example, including race in algorithms that predict how much longer a person would live after a kidney transplant may fail to account for individual circumstances that could limit how much longer they live.
Unanswered questions and future work
Better biomarkers may one day be able to better predict health outcomes than race and ethnicity. Until then, including race in certain clinical algorithms could help reduce disparities.
Although my study uses an equality of opportunity framework to measure how race and ethnicity affect the results of prediction algorithms, researchers don’t know whether other ways to approach fairness would lead to different recommendations. How to choose between different approaches to fairness also remains to be seen. Moreover, there are questions about how multiracial groups should be coded in health databases and algorithms.
My colleagues and I[24] are exploring many of these unanswered questions to reduce algorithmic discrimination. We believe our work will readily extend to other areas outside of health, including education, crime and labor markets.
References
- ^ increasingly concerned (doi.org)
- ^ estimated glomerular filtration rate, or eGFR (doi.org)
- ^ allocate kidney transplants in the U.S. (optn.transplant.hrsa.gov)
- ^ trained on actual GFR values from patients (www.kidney.org)
- ^ lead to larger discrepancies (doi.org)
- ^ health economist and statistician (scholar.google.com)
- ^ could worsen health inequities (www.science.org)
- ^ different economic frameworks (plato.stanford.edu)
- ^ utilitarian outlook (doi.org)
- ^ equality of opportunity (www.jstor.org)
- ^ political science (www.jstor.org)
- ^ economics (press.uchicago.edu)
- ^ law (www.jpe.ox.ac.uk)
- ^ several landmark rulings in education (edeq.stanford.edu)
- ^ SDI Productions/E+ via Getty Images (www.gettyimages.com)
- ^ violent and unsafe environments (theconversation.com)
- ^ limited access to healthy food (theconversation.com)
- ^ accountable effort (doi.org)
- ^ evaluate whether race should be included (www.science.org)
- ^ race is a social and not biological construct (www.genome.gov)
- ^ proxies for various circumstances (www.ama-assn.org)
- ^ increase systemic inequities and discrimination (www.science.org)
- ^ can also increase disparities (www.science.org)
- ^ My colleagues and I (sop.washington.edu)
Authors: Anirban Basu, Professor of Health Economics, University of Washington